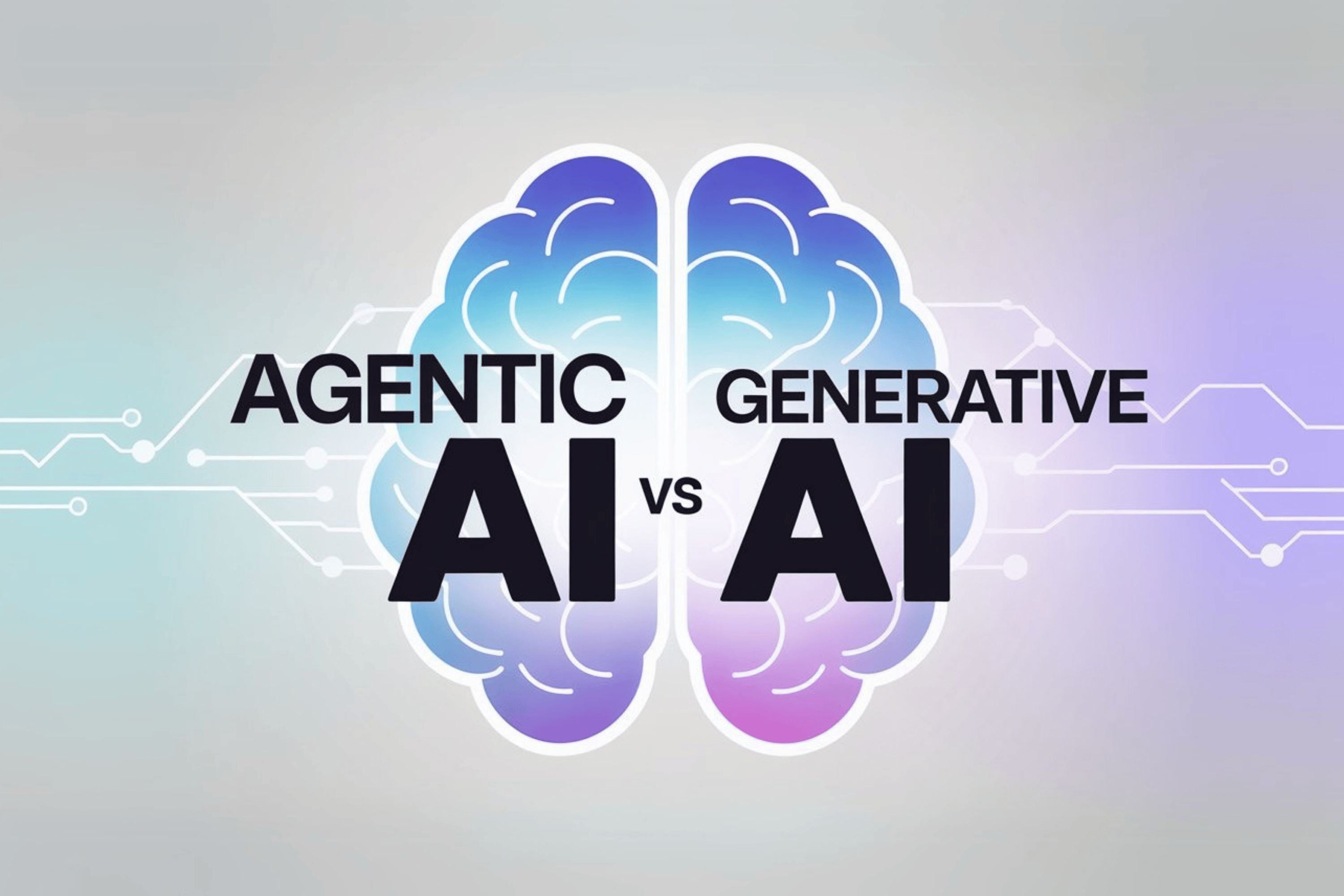
We once directed AI. Now, it co-authors our decisions.
Generative AI is now writing content, designing visuals, and speeding up creative workflows. It's impressive. But another kind of AI is quietly stepping onto the scene. Agentic AI goes far beyond content creation. It can research, plan, and carry out complex tasks across entire workflows with little to no supervision.
According to Gartner, nearly 30 percent of operational processes will rely on agentic systems by 2025. These aren't tools waiting for prompts. They're systems taking action.
Understanding the difference between generative and agentic AI isn’t just helpful. It’s essential to making the right strategic calls.
Defining the Technologies: What Are We Really Talking About?
What is Generative AI?
Generative AI refers to systems designed to create new content based on patterns learned from training data. These models excel at:
- Producing human-like text, images, audio, or video
- Transforming one type of content into another
- Creating variations on existing content
- Responding to specific prompts with relevant outputs
At its core, generative AI predicts what should come next in a sequence—whether that's the next word in a sentence, pixel in an image, or note in a melody. Models like GPT-4, DALL-E, and Midjourney fall into this category, serving primarily as sophisticated content generation tools.
What is Agentic AI?
Agentic AI represents a significant evolution beyond mere generation. These systems can:
- Set and pursue goals independently
- Make decisions across multiple steps
- Use tools and APIs to interact with other software
- Maintain memory of past actions and results
- Adapt strategies based on outcomes
- Self-evaluate and correct course as needed
Rather than simply responding to prompts, agentic AI actively works toward objectives, making decisions about what actions to take next. This autonomy allows these systems to handle complex workflows with minimal human supervision.
The Architectural Distinction: How They're Built Differently
Under the Hood of Generative AI
Generative AI typically follows a relatively straightforward architecture:
- Input processing: Receives and interprets user prompts
- Token prediction: Generates tokens (words, image elements, etc.) based on statistical probability
- Output assembly: Constructs cohesive content from these predictions
- Delivery: Returns the finished product to the user
These systems operate within a single context window and generally have limited awareness beyond the immediate generation task.
The Complex Architecture of Agentic Systems
Agentic AI incorporates additional components that enable goal-directed behavior:
- Planning module: Breaks down objectives into actionable steps
- Memory management: Maintains information across multiple interactions
- Tool integration framework: Connects with external software and resources
- Decision engine: Evaluates options and selects next actions
- Self-assessment mechanism: Monitors progress and adjusts approach
- Feedback processing: Learns from successes and failures
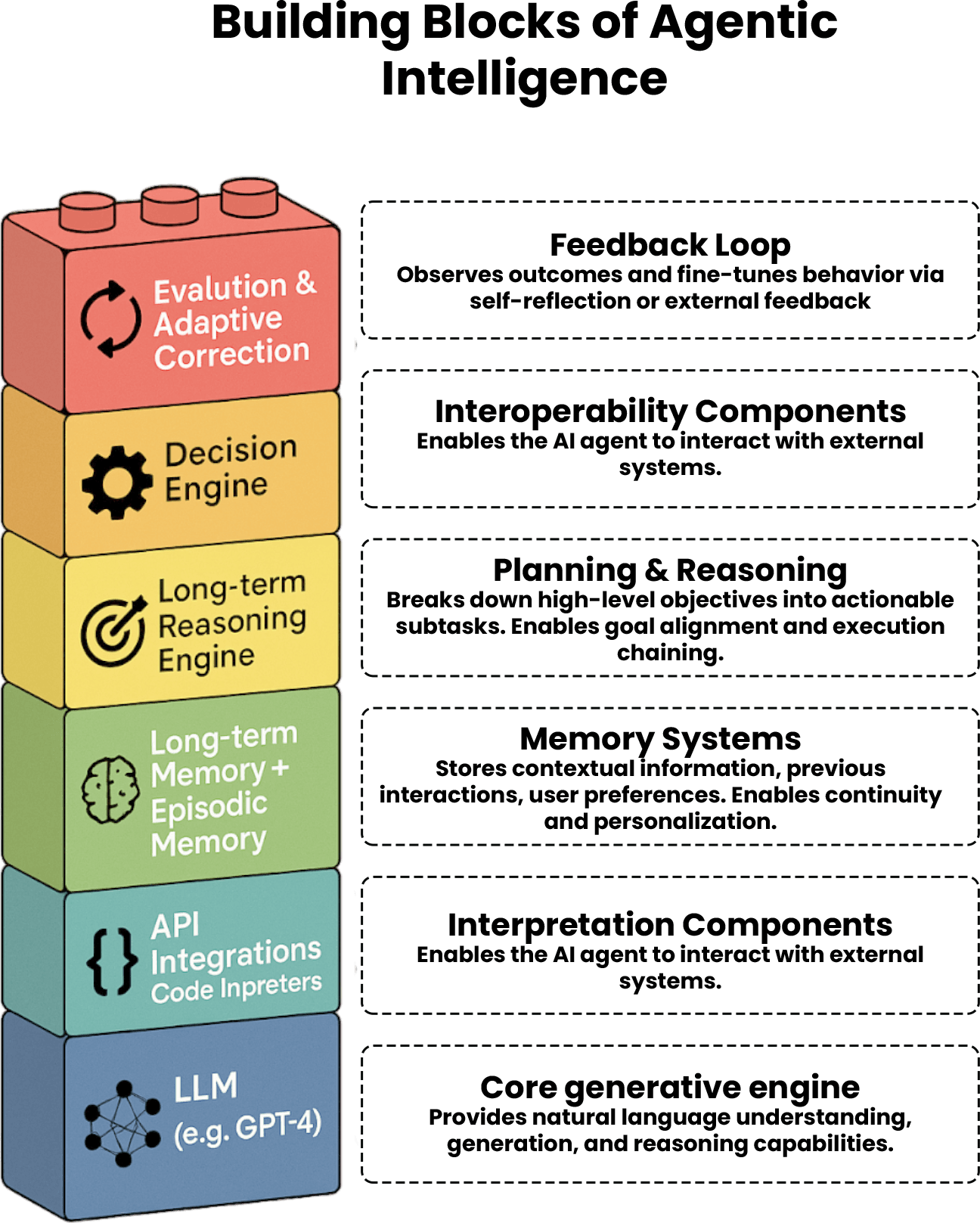
This architecture enables agentic AI to operate with greater independence and tackle more complex tasks that require sustained attention and adaptability.
Comparative Analysis
Feature | Generative AI | Agentic AI |
---|---|---|
Primary function | Creates content | Completes tasks |
Autonomy level | Reactive, prompt-driven | Proactive, goal-driven |
Decision scope | Limited to immediate output | Extended across multiple actions |
Memory capability | Confined to context window | Persistent task memory |
Tool usage | Limited or none | Extensive, purpose-selected |
Human interaction | Direct instruction | Goal-oriented supervision |
Error handling | Human correction required | Self-correction capable |
This fundamental difference in design leads to distinctly different capabilities and ideal use cases.
Optimal Use Cases
When to Deploy Generative AI
Generative AI excels in scenarios focused on content creation and transformation:
- Marketing content generation (blog posts, ad copy, social media)
- Creative assistance for writers, designers, and developers
- Data augmentation for training other AI systems
- Content personalization at scale
- Language translation and content adaptation
- Brainstorming and ideation support
The value proposition centers on amplifying human creative capabilities and reducing time spent on routine content production.
When to Leverage Agentic AI
Agentic AI delivers maximum value for complex, multi-step processes:
- Research assistance that spans multiple sources and synthesizes findings
- Customer service automation that can navigate complex troubleshooting paths
- Process optimization with the ability to monitor, analyze, and suggest improvements
- Project management assistance that tracks progress and coordinates actions
- Data analysis that identifies patterns and proactively generates insights
- Continuous learning systems that improve through accumulated experience
These systems shine when tasks require sustained attention, adaptation, and the coordination of multiple tools and information sources.
Conclusion: Making Strategic Choices
The choice between generative and agentic AI isn't necessarily an either/or proposition. Many organizations will benefit from deploying both technologies, with generative AI handling content creation tasks while agentic systems tackle more complex, multi-step processes.
The key is understanding the fundamental differences in how these technologies work and what they do best. With this knowledge, you can make informed decisions about:
- Which AI approach fits specific business challenges
- How to integrate these technologies into existing workflows
- What skills your team needs to effectively work alongside AI
- Where to focus AI investments for maximum impact
As these technologies continue to evolve, the organizations that thrive will be those that strategically apply both generative and agentic capabilities to enhance human potential rather than simply replace it.