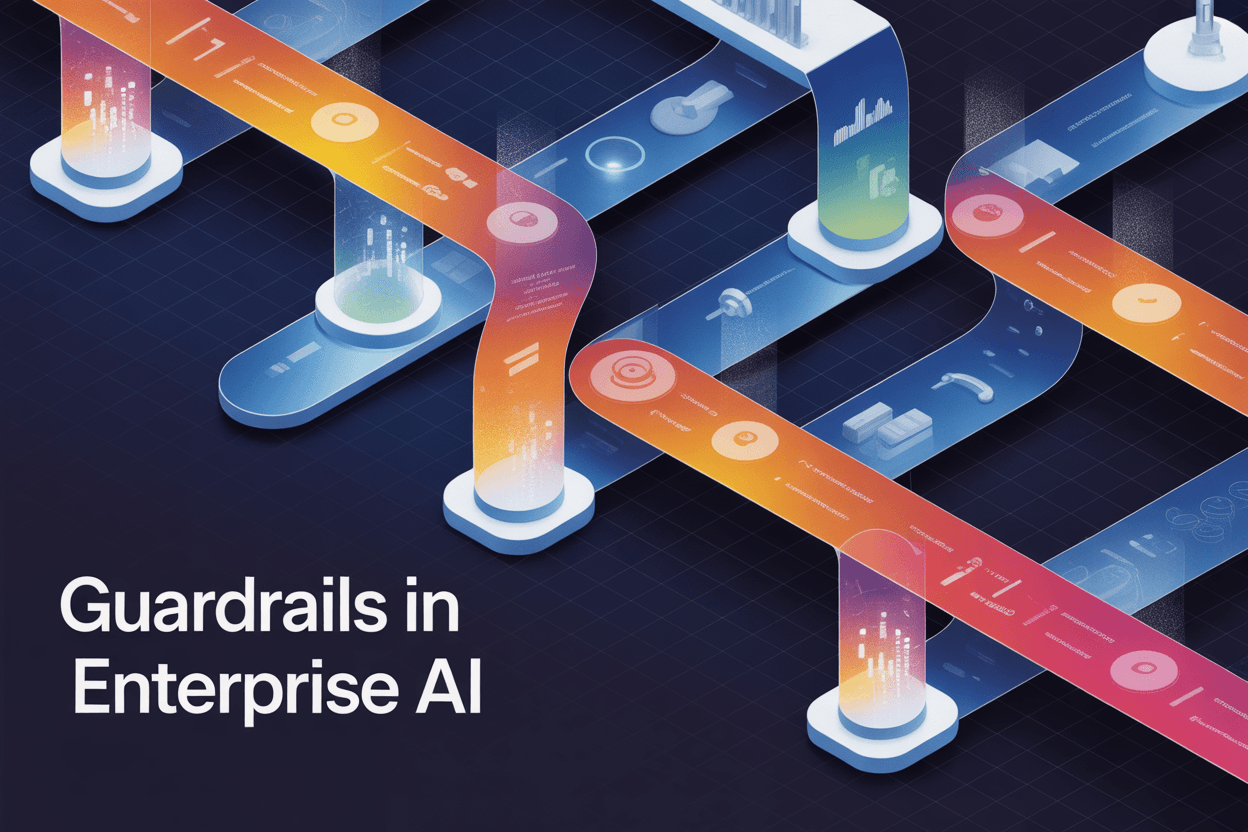
Responsible AI (RAI) is becoming a top priority for companies that use artificial intelligence. As they start using Agentic AI tools like Zigment.ai, they need to handle ethical concerns such as data security, bias in algorithms, and following regulations. This framework explains how Responsible AI principles—covering things like governance, transparency, and risk management—help businesses use AI in an ethical way and avoid issues like data leaks or breaking rules. By following standards like ISO 27001 and AICPA SOC, companies can make Responsible AI a competitive advantage, build trust with stakeholders, and keep their AI systems secure, clear, and accountable. More and more organizations see that being responsible with AI isn’t just about avoiding risks—it’s also about creating lasting business value through trustworthy AI systems that customers and partners can rely on.
What Is Responsible AI?
Responsible AI refers to the development and deployment of artificial intelligence systems that prioritize ethical values, security, fairness, and transparency. These systems are designed with safeguards to protect user data while delivering reliable, unbiased results. Enterprise AI requires specialized frameworks that account for issues like algorithmic bias, data protection, and system explainability. Without proper governance, AI systems can expose proprietary data, produce misleading outputs, or violate regulatory requirements.
The concept extends beyond technical implementation to encompass organizational culture, processes, and governance mechanisms that ensure AI systems operate within ethical boundaries. Unlike consumer applications, enterprise AI often processes highly sensitive information across complex workflows, increasing both the potential benefits and risks. Organizations implementing Responsible AI frameworks must balance innovation with appropriate controls, ensuring their systems can be trusted by all stakeholders.
The Three Pillars of Enterprise-Responsible AI
1. Data Security: Protecting Proprietary Information
Data security is a critical concern in enterprise AI adoption, especially with Large Language Models (LLMs) that handle sensitive information. AI-driven data leakage can expose confidential information through outputs, particularly with generative AI technologies that might reconstruct training data in their responses. Organizations should align their security practices with frameworks like ISO 27001, which offers standardized risk assessment methodologies and structured data protection protocols.
Effective data security for AI systems requires specialized approaches beyond traditional data protection. Organizations must implement prompt engineering techniques that prevent sensitive data extraction, deploy robust authentication systems, and establish clear data retention policies for model training and inference. Leading enterprises employ techniques like differential privacy and federated learning to preserve utility while minimizing exposure risks.
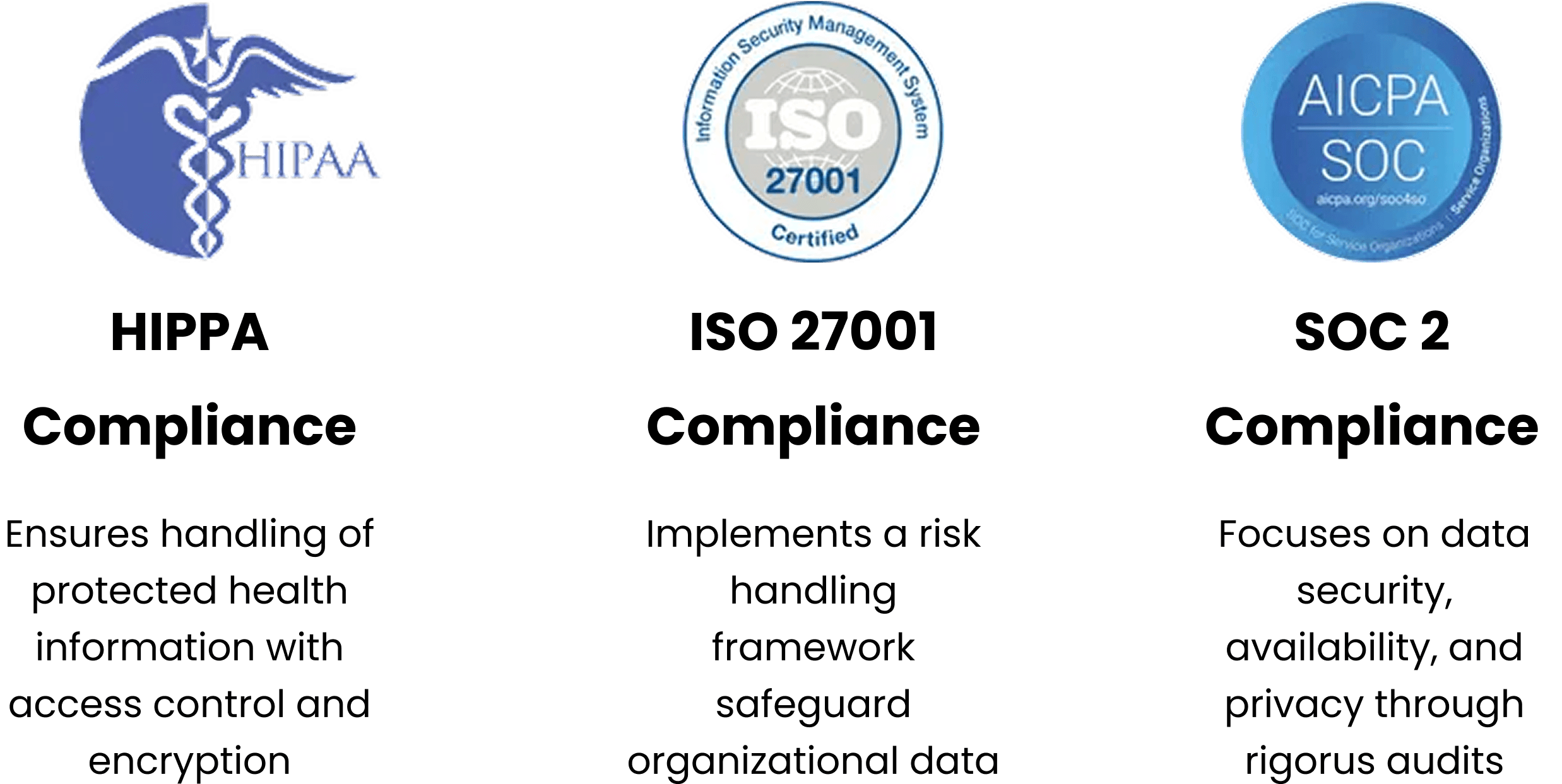
2. Trustability: Ensuring Policy Compliance
Trustability focuses on ensuring AI systems operate reliably within defined parameters and produce accurate, dependable results. Establishing effective AI governance frameworks incorporates Responsible AI principles into existing information security systems, creating a unified approach to risk management. Trustable AI systems maintain performance across diverse inputs and operate consistently with organizational values and regulatory requirements.
Guardrails are necessary to prevent AI "hallucinations"—instances where models generate incorrect outputs that appear plausible but contain fabricated information. Techniques include input validation, output filtering, confidence scoring systems, and human review processes for high-stakes decisions. Organizations must develop clear thresholds for when AI outputs require additional verification or human oversight.
AICPA SOC certification aligns with trustability requirements, providing assurance on security controls, system availability, processing integrity, confidentiality protections, and privacy safeguards. This certification demonstrates to stakeholders that AI systems meet established standards for trustworthy operation.
3. Observability: End-to-End Traceability
Observability enables organizations to understand AI systems' operations and decisions throughout their lifecycle. Implementing comprehensive traceability requires tracking data flows and model decisions across the AI pipeline, from data collection through model training to inference and outcome evaluation. Observability supports continuous improvement, regulatory compliance, and timely intervention when systems behave unexpectedly.
Modern observability frameworks incorporate model monitoring dashboards, data lineage tools, and automated alerting systems that flag potential issues before they impact business operations. Organizations implementing robust observability can trace specific outputs back to their inputs, understand which features influenced decisions, and identify potential sources of bias or performance degradation.
Real-time monitoring strategies, such as performance dashboards and anomaly detection, are crucial for effective observability. Healthcare organizations must also ensure HIPAA compliance while providing necessary audit trails that track who accessed sensitive information and how AI systems processed protected health data.
What Is Enterprise AI Governance?
AI governance encompasses frameworks, policies, and oversight mechanisms guiding AI development and deployment across complex organizational structures. Unlike consumer AI applications, enterprise AI requires governance approaches that account for regulatory requirements, industry standards, and business risk profiles. Organizations should adopt a phased approach to implementation:
- Foundation Phase: Establish baseline governance structures aligned with ISO 27001 and AICPA SOC requirements. This includes defining clear roles and responsibilities, implementing risk assessment methodologies, and creating initial AI policies that guide development efforts.
- Integration Phase: Incorporate AI safeguards into existing security frameworks, focusing on data leakage prevention and model security. During this phase, organizations connect AI governance with broader information security practices, creating unified approaches to managing digital risks.
- Maturity Phase: Develop advanced monitoring capabilities and continuous improvement mechanisms that adapt to evolving threats, regulatory changes, and business needs. Mature governance frameworks incorporate feedback loops from multiple stakeholders and leverage metrics to drive ongoing enhancements.
Key Components of Effective AI Governance:
- Policy Development: Balance innovation with controls for AI deployment through clear guidelines that address model selection, data usage, and deployment criteria.
- Review Processes: Structured reviews for technical and ethical compliance that scale based on risk levels and potential impacts.
- Documentation Requirements: Comprehensive tracking of datasets, models, and testing procedures that supports audits and demonstrates compliance.
How Responsible AI Mitigates Organizational Risks
Responsible AI directly addresses critical challenges enterprises face in their AI implementation journeys. By embedding ethical considerations and control mechanisms throughout the AI lifecycle, organizations can avoid significant pitfalls:
- Regulatory Penalties: Non-compliance with laws like the EU AI Act can result in fines reaching 6% of global annual revenue, creating significant financial risk. Responsible AI frameworks incorporate regulatory requirements into development processes, reducing compliance gaps.
- Reputational Damage: AI systems that produce biased, harmful, or misleading outputs can severely damage brand trust and customer relationships. By implementing appropriate guardrails and testing protocols, organizations prevent these reputation-damaging incidents before they occur.
- Operational Disruptions: Failed AI implementations or models that produce unreliable results can disrupt critical business operations. Real-time monitoring and observability practices identify potential issues early, minimizing business impact.
The Responsibility of Developers Using Generative AI
Developers working with generative AI technologies face unique challenges and responsibilities due to these systems' powerful capabilities and potential for misuse. Responsible implementation requires specific technical approaches:
- Preventing Data Leakage: Use differential privacy techniques that add calculated noise to training data, federated learning approaches that keep sensitive data local, and robust output filtering to prevent exposure of proprietary information.
- Implementing Guardrails: Create comprehensive systems that validate inputs for potentially harmful content, filter outputs that might violate organizational policies, and implement confidence scoring to flag uncertain predictions for human review.
- Maintaining Oversight: Conduct regular security assessments of AI systems, perform bias audits across diverse demographic groups, and implement continuous monitoring that tracks model performance in production environments.
Implementing Fair and Responsible AI for Consumers
Creating AI systems that treat end users ethically requires specific design considerations focused on transparency, control, and feedback mechanisms:
- Transparency: Explain data usage and AI decision-making in plain language that diverse users can understand. Organizations should provide appropriate levels of detail without overwhelming users with technical information, focusing on what matters most for informed consent.
- Control Mechanisms: Provide intuitive interfaces that let users review, correct, or opt out of AI-driven decisions. Effective control systems balance ease of use with meaningful options that give consumers genuine agency over how AI affects their experiences.
- Feedback Channels: Create clear pathways for consumers to report concerns about AI systems and resolve issues quickly. Organizations should analyze aggregated feedback to identify systemic problems and implement improvements based on user experiences.
How Zigment Demonstrates Responsible AI
Zigment.ai exemplifies responsible AI through its marketing and sales automation platform by integrating ethical principles throughout its operations:
- Fairness in Data Handling: Using diverse datasets minimizes biases.
- Transparent Processes: Clear explanations of AI operations build client confidence.
- Privacy Protection: Advanced encryption and strict protocols ensure compliance.
- Continuous Monitoring: Ongoing assessment enables bias identification.
- User Control: Preference management tools empower consumers.
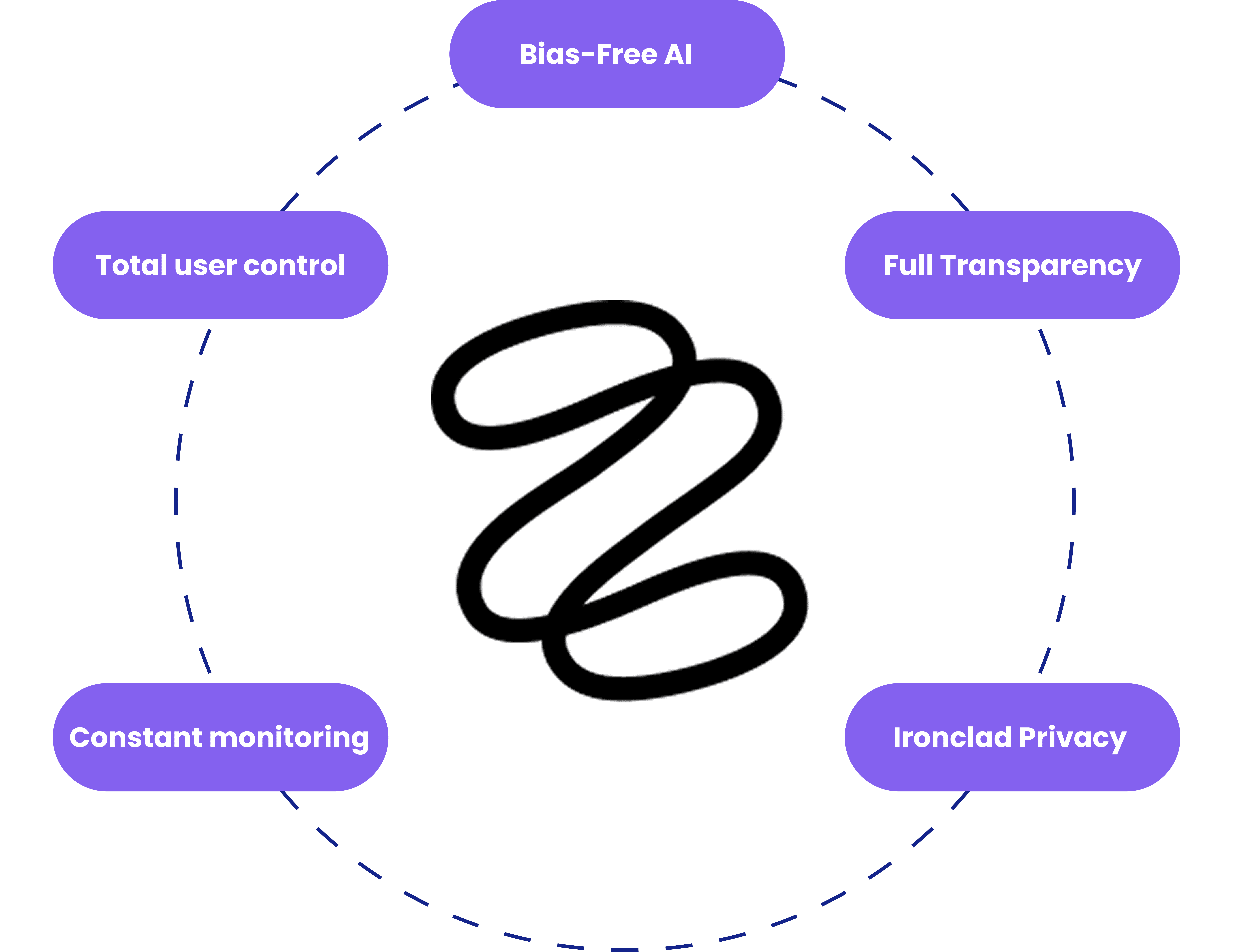
Zigment's Responsible AI for Enterprises
Conclusion: Responsible AI as Competitive Advantage
Implementing responsible AI across the three pillars of data security, trustability, and observability positions organizations for sustainable success in an increasingly AI-driven world. Zigment.ai emphasizes that embracing Responsible AI principles leads to personalized customer experiences, optimized operations, and lasting trust—creating significant competitive advantages in crowded markets.
Organizations that treat responsible AI as a strategic imperative rather than a compliance burden can unlock greater value from their AI investments while avoiding costly pitfalls. By integrating responsible AI with established certifications like ISO 27001 and AICPA SOC, enterprises create a foundation for ethical, secure, and compliant AI deployment that meets stakeholder expectations while driving innovation.
As AI capabilities continue to advance, the organizations that thrive will be those that implement these technologies in ways that earn and maintain trust across their entire ecosystem of customers, partners, and regulators.