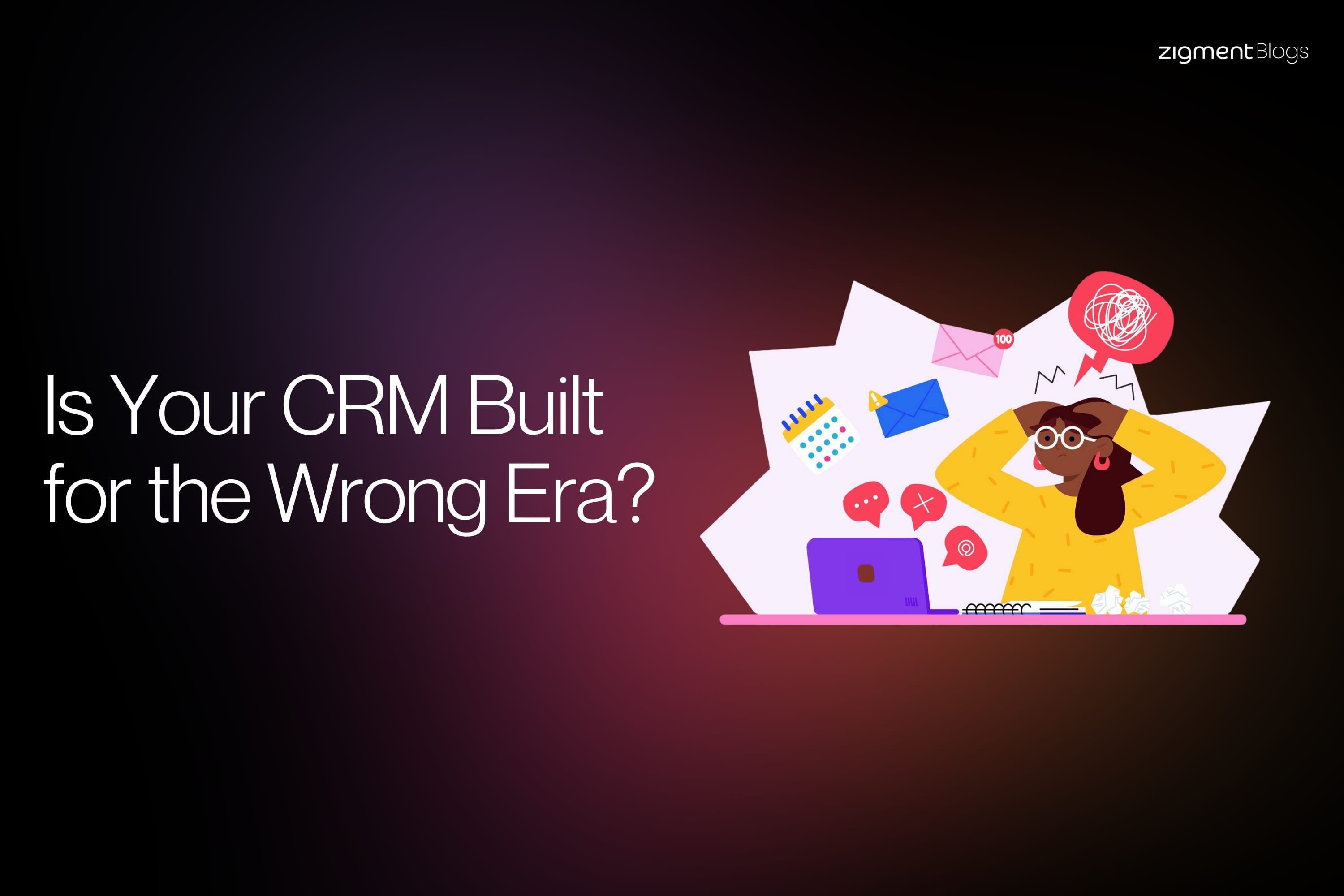
The cracks in the legacy CRM model
Customer‑relationship management platforms were born in an era when marketing channels were few, data volumes were modest, and every new record was typed in by a human. The CRM became the single “system of record,” a centralized ledger of names, phone numbers, emails, tasks, and notes. For years that paradigm served its purpose: sales reps could retrieve a prospect’s history, marketing teams could export a list for the next campaign, and managers could run pipeline reports.
Yet the modern marketing stack has exploded far beyond the CRM’s original design brief. Paid‑media dashboards, chat widgets, call‑tracking tools, website analytics, product‑usage logs, and support ticketing systems all generate their own streams of customer data—most of which live in silos that barely talk to one another. Stitching that information into a coherent picture of the customer journey is now one of the biggest operational headaches in growth‑oriented companies.
The fragmented customer journey
The gap becomes painfully obvious whenever you try to answer a seemingly simple question: What happened to the leads from last month’s webinar? You may find the registrations in a marketing‑automation platform, the follow‑up emails in a different tool, the sales calls logged in the CRM (if the rep remembered to hit “save”), and the closed‑won deals in an invoicing system. Each application holds a shard of the truth, but no single system captures the narrative from first click to loyal customer.
Human-driven inconsistencies
Even within the CRM itself, data quality varies wildly because it still relies on people to update fields, log activities, and tag opportunities. Some reps are meticulous; others forget, get busy, or invent their own naming conventions. The result is a patchwork record that explains why two companies using the same CRM can experience vastly different outcomes.
Deterministic data vs. conversational insight
The limits of countable metrics
Legacy CRMs also reflect a deterministic view of the funnel. Most fields describe countable events or timestamps: how many emails were sent, the date a call occurred, the size of a deal, or the stage of an opportunity. Those metrics matter, but they miss the nuance now unfolding inside AI‑driven conversations. When an intelligent chatbot negotiates pricing, qualifies a lead, or handles an objection, the richest insights are embedded in the dialog itself—the phrases a prospect uses, the hesitation before clicking a link, the sentiment that shifts from skepticism to excitement. None of that fits neatly into the old tabular schema of “Activity Type” and “Date/Time.” If we continue to store only deterministic breadcrumbs, we lose the context that makes agentic interactions so powerful.
The rise of conversational context
In the Agentic AI era, the conversation is quickly becoming the primary data asset. AI agents can run qualification interviews, provide product demos, recommend next steps, and schedule follow‑ups—all without human intervention. Every sentence exchanged and every micro‑decision made along the way carries signal about buyer intent, objections, and emotional readiness. An AI‑first CRM must therefore treat conversational data as a first‑class citizen. That means capturing transcripts, embeddings, sentiment scores, and decision paths in a structure that allows other agents—or humans—to query, summarize, and act on that information in real time.
Rethinking the architecture of CRMs
Doing so calls for a radical redesign of the system of record. Instead of static tables labeled “Leads,” “Contacts,” and “Deals,” picture a living, flexible data model where hard facts and probability‑based insights sit side by side. Each record can store not only when an email was sent but also the language model’s confidence score that the recipient is price‑sensitive; not only that a call happened but also the emotional trajectory of the caller extracted from voice analysis; not only the number of website visits but also the sequence of page scrolls that predicted an 80 percent likelihood of conversion. These data points are high‑volume, high‑velocity, and often non‑deterministic, meaning they represent probabilities rather than certainties. Traditional relational databases strain under that complexity. New‑age AI‑first platforms leverage modern data techniques with completely reimagined data store design and real‑time analytics layers to keep everything query-able without sacrificing performance.
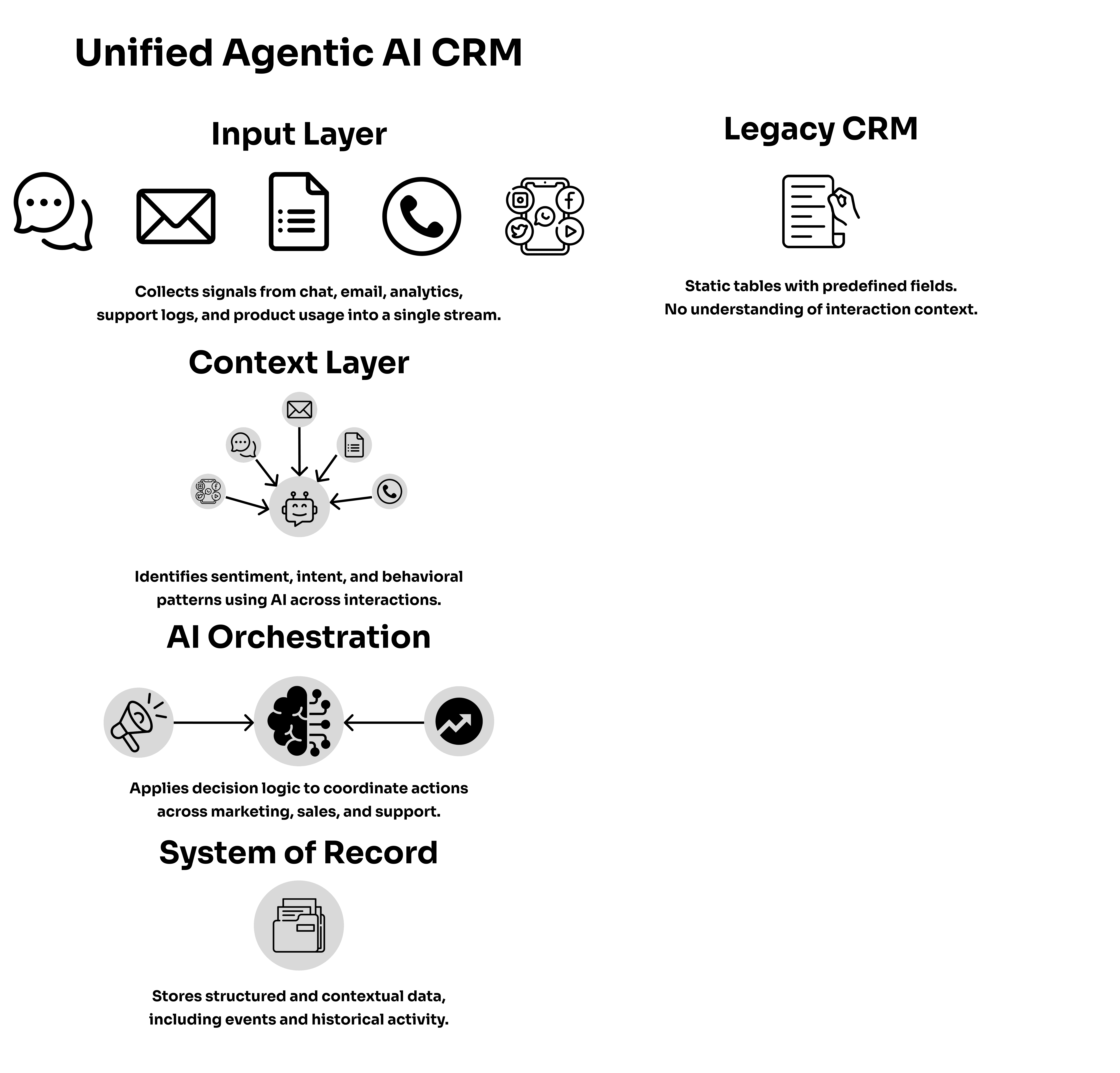
Seeing the whole funnel through Agentic AI
Because the agent itself performs many actions that humans once handled, the platform sees a far broader slice of the funnel than any single department ever could. A marketing AI can adjust ad bids, rewrite landing‑page copy, and route promising visitors to a sales AI that books demos. The entire choreography is logged by the platform, providing a panoramic view of the journey that older CRMs simply never captured. That breadth is a competitive advantage: the more surface area an agentic platform observes, the better its models become at predicting which engagements move the needle and which are noise.
Legacy vs. AI‑first platforms
Below is a visual comparison of legacy CRMs and AI‑first marketing platforms:
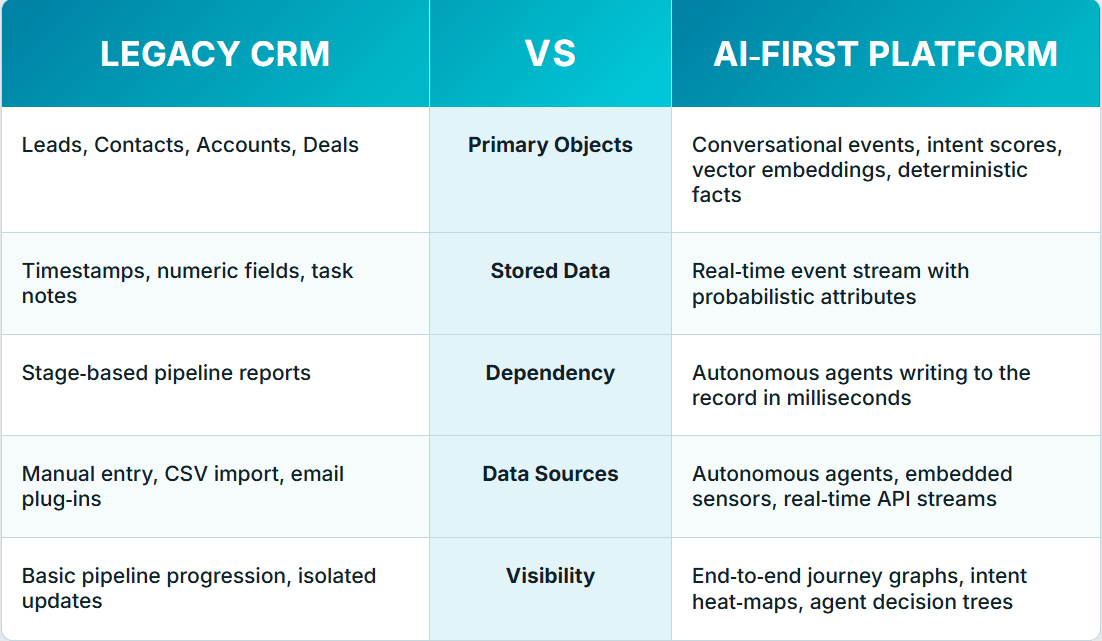
Operational advantages of AI-first systems
Real-time responsiveness
As more funnel activities shift to AI agents, companies that adopt an AI‑first system of record will benefit from faster feedback loops. Instead of waiting for a weekly meeting to discover that webinar leads are stagnating, an agentic platform can notice the trend in minutes and trigger a new nurture path, update ad targeting, or alert a human when nuanced intervention is needed. Because the underlying data layer already contains conversational context, the next agent—whether marketing, sales, or support—starts with full situational awareness. That continuity is impossible when data is fragmented across a dozen tools and updated by fallible humans.
Built-in compliance and efficiency
Critics may argue that storing every conversational detail will create data bloat and complicate compliance. AI‑first vendors are addressing those concerns by embedding privacy filters, PII redaction, and retention policies directly into the data pipeline. They also leverage semantic compression, storing vector representations instead of raw transcripts when appropriate, so queries remain efficient. Moreover, the ability to answer regulatory questions—“Show me every interaction in which a customer asked about data usage”—actually improves when the platform maintains a complete, searchable event history.
A transition, not a tear-down
The transition will not happen overnight. Many organizations have invested millions in customizing their existing CRMs, and ripping them out is unrealistic. Instead, forward‑looking teams are deploying AI‑first marketing platforms alongside their legacy systems. The new platform becomes the engagement layer and real‑time brain, while the old CRM continues to serve as a compliance archive or billing back‑end. Over time, as confidence grows and use cases expand, the AI‑first record gradually assumes center stage.
The future of the system of record
In the 1990s a CRM was revolutionary because it centralized rolodexes and sticky notes. In the 2000s integrations and APIs made it a hub for email and call logs. Today the revolution is conversational and probabilistic, driven by agents that learn and act continuously. To harness that power, we must rethink what it means to be a “system of record.” The next generation will not merely store who did what and when; it will capture why decisions were made, how prospects felt, and which conversational cues predicted success. Those insights will fuel even smarter agents, closing the loop between data and action in ways legacy CRMs were never built to handle.
Why the shift matters now
Performance marketers, growth leaders, and RevOps teams who embrace this shift will gain unprecedented visibility and agility. Those who cling to deterministic schemas and manual data entry will struggle to keep pace with AI‑driven competitors. The future of customer data is granular, conversational, and agentic—and the time to redesign our systems of record is now.